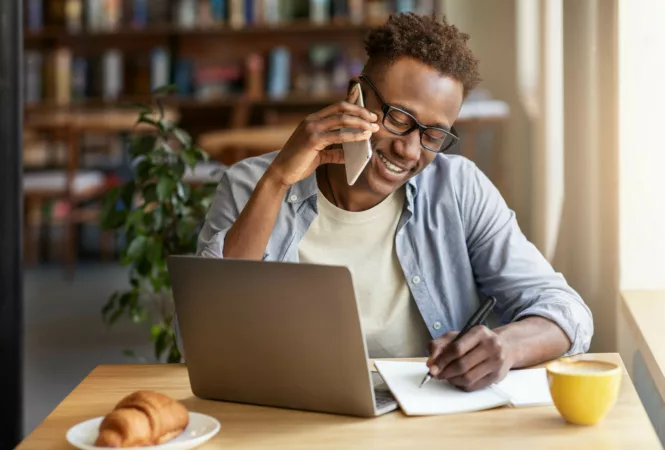
The Local Marketer’s Introduction to Sentiment Analysis
The modern marketer relies on a treasure trove of data at their fingertips: from starred reviews on business profiles to social media performance metrics, we are no longer in the dark about what our customers think, how they feel, or ultimately, how they tend to behave.
While amazing, that mass of data poses another massive challenge. With all this raw information coming in at a mile a minute, how can local marketers make sense of it for bigger moves and tactical deployments in their local marketing strategies?
Thankfully, marketing tech stacks exist to assist with analyzing data and inform next steps across the user journey, from how to target a “try us” campaign to setting up loyalty programs. While quantitative feedback like net promoter scores can be useful, qualitative feedback such as text-based insights offer a potential goldmine—if you can comb out the insights from all that unstructured data. That is why sentiment analysis affords one of the biggest and most useful tools in the marketer’s arsenal these days.
What is Sentiment Analysis?
At its most basic level, sentiment analysis looks at what people are saying about a subject—in this case, a business or a location—and works out whether they’re saying something good, bad, or indifferent.
More detailed, sentiment analysis examines the views or opinions of people who came into contact with something. It’s a way to determine the opinions, judgments, or emotions behind the “natural language”, or the words real people actually use, even in larger sample sets. (Of course, meaning is conveyed through more than just the words, such as the overall context, body language, and more—in the case of digital language, those include emojis, gifs, punctuation, and other non-verbal markers).
What are Sentiment Analysis Tools?
Sentiment analysis tools are software applications or platforms designed to analyze text data and determine the sentiment expressed within it. Specifically, they employ techniques such as natural language processing, machine learning, statistical analysis and lexicon-based analysis to classify the sentiment of text as positive, negative, or neutral.
How Do Sentiment Analysis Tools Work—In Theory
Modern technology makes digital sentiment analysis much more accessible. In fact, efficient sentiment analysis at scale emerged as a main driver of multi-location businesses adopting AI models, by harnessing machine learning techniques and using natural language processing.
Digital tools also save a human having to go through every single mention of your brand, parsing what the person behind the comment actually means to say, and categorizing them—a process that also tends to bring that person’s own biases to the categorization, too.
What Goes Into Sentimental Analysis?
With sentiment analysis, businesses can take all of that raw data from customers—all the reviews, social media mentions, feedback forms, surveys, emails, and so on—then run it through algorithms designed to spot patterns and extract information. If you’ve ever filled in a survey or left a review on Facebook, chances are your words have been crunched via sentiment analysis. This enlarged scale for crunching data even led some people to refer to sentiment analysis as “opinion mining”.
Specifically, sentiment analysis tools categorize text into three primary sentiment polarities:
Positive sentiment indicates overall favorable feelings.
Negative sentiment denotes dissatisfaction or frustration.
Neutral sentiment doesn’t lean either way with typically factual statements.
Categorizing these manually would be time-consuming, nigh on impossible to keep up with, especially for smaller marketing teams or local marketers. That’s where sentiment analysis tools come in, turning the unstructured data into useful insights at the click of a button, tracking incoming data in real-time, too, so you can spot emerging trends or issues early.
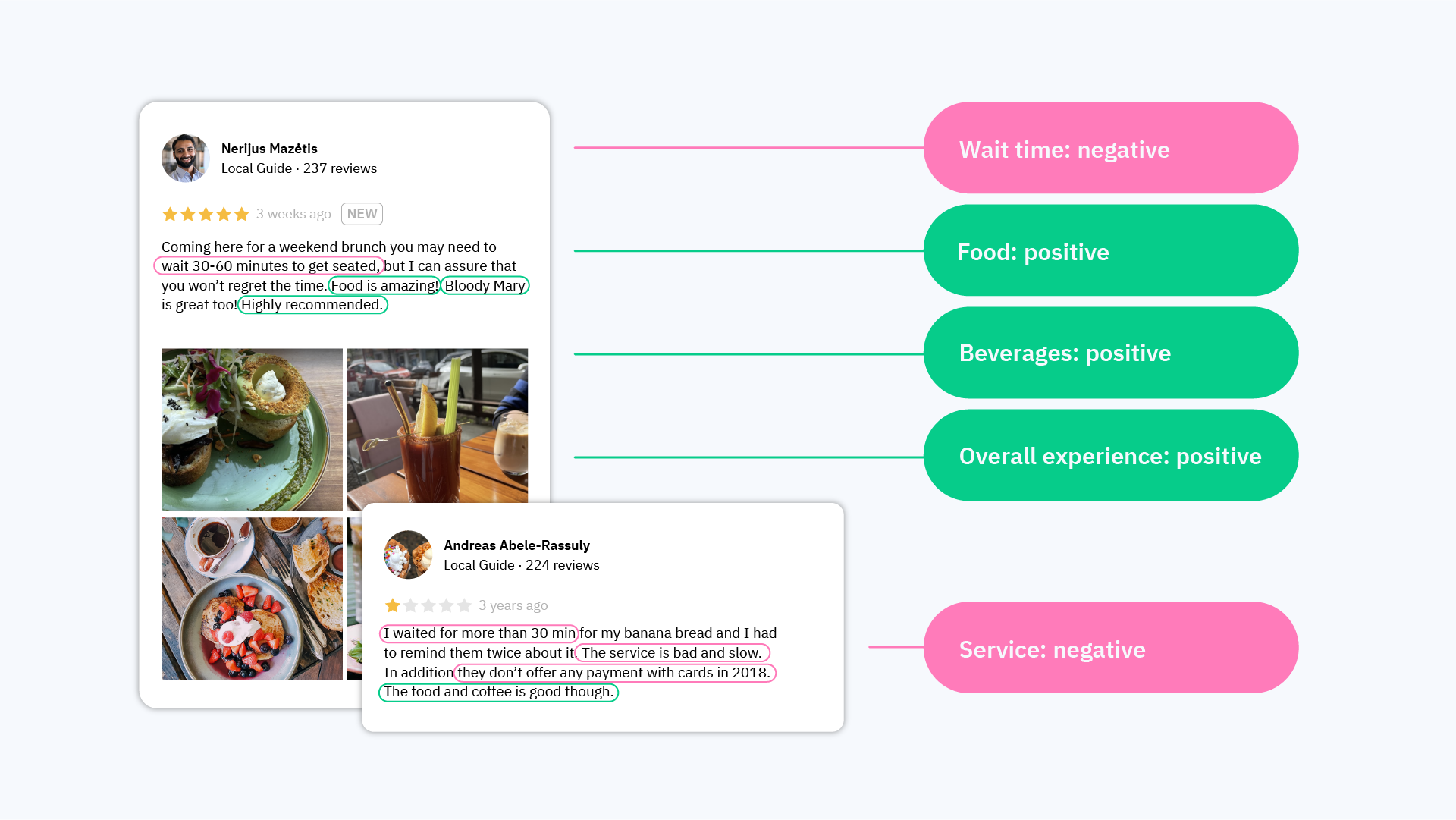
Behind the scenes, sentiment analysis either builds on:
rules-based algorithms that assign scores to words
machine learning models trained on natural language feedback
Rules-Based Algorithms
Rules-based algorithms assign scores to words based on predefined rules or dictionaries. Each word is evaluated individually, and its sentiment score contributes to the overall sentiment of the text.
For example, words like "happy" or "love" might be assigned positive scores, while words like "sad" or "hate" might be assigned negative scores. The sentiment of the entire text is then calculated based on the aggregate scores of its constituent words.
Machine Learning Models
Machine learning models are trained on large datasets of natural language feedback, where the sentiment of the text is labeled by humans (e.g., positive, negative, neutral). The model learns to recognize patterns and associations between words and sentiments during the training process.
Once trained, the model can predict the sentiment of new, unseen text based on the patterns it has learned from the training data. These models often utilize techniques such as neural networks, support vector machines, or decision trees to make predictions about the sentiment of text data.
Rev Up Your Growth With Digital Visibility
How Do Sentiment Analysis Tools Work—In Practice
Let’s look at two front-end examples of how location-based marketers can use sentiment analysis tools, and how those work in situ.
Social Media Sentiment Analysis
Social media mining is one of the most accessible areas for discovering what people are saying about their experience with your brand. Users are generally off-the-cuff and less inhibited on social media, more likely to share their perceived experiences, both good and bad, or even neutral. Yet, 70% of companies ignore this massive opportunity to understand what lies behind customer complaints on social media.
EXAMPLE: Imagine launching a new product and announcing it on social media platforms like Twitter, Facebook, and Instagram. Social media sentiment analysis tools would monitor the comments, mentions, and posts related to the product across these platforms. For instance, if users tweet, "Just got my hands on the new XYZ, and I love it! #amazing #bestpurchase," the sentiment analysis would recognize these positive sentiments. Conversely, if another user posts, "Disappointed with the battery life of the new XYZ. Not worth the hype," the sentiment analysis would identify negative sentiments. By analyzing the overall sentiment across social media, you can gauge public perception, identify areas for improvement, and tailor your marketing or product strategies accordingly.
Reviews Management Sentiment Analysis
Whether you’ve prompted a customer to leave a review as part of the sales journey, or they’ve taken it upon themselves, customer reviews can be used to identify gaps and pain points. Sentiment analysis for review management can also inform in-store promotions and improvements to positive experiences. The process here works in a similar way to social media sentiment analysis: scraping the web, looking for reviews of your store or brand, then running all these through the analysis tool to extract keywords, visualize the data, plus assigning categories and tags to the text.
EXAMPLE: Consider receiving numerous online reviews on platforms like TripAdvisor, Yelp, or Google Reviews. Review management sentiment analysis tools help you analyze the sentiments expressed in these reviews to understand customer satisfaction levels. If a guest writes, "Exceptional service and comfortable rooms. Will definitely stay here again," the sentiment analysis would recognize the positive sentiment. Conversely, if another guest leaves a review stating, "Poor customer service and cleanliness issues in the rooms," the sentiment analysis would identify negative sentiments. By aggregating and analyzing these reviews, you can make improvements to enhance guest satisfaction and reputation.
Why Should Businesses Care About Sentiment Analysis?
Yes, people are out there talking about your business, your locations, your staff, right now. What they say matters: electronic word of mouth (eWOM) has become one of the strongest indicators of social proof for today’s businesses. People listen to people, and 90% of consumers read online reviews before visiting a business, while 84% trust online reviews as much as personal recommendations.
You might not always think of people forming an “emotional connection” to a brand, but every interaction does elicit an emotion of some kind. Marketers need to understand those emotions, so they can know how their brand performs, and whether they need to improve anything to continue growing their business. Customer feedback remains king, and sentiment analysis marketing empowers more effective data-driven decision-making.
Analysis includes more than just finding out what people say about their experiences with your brand. Sentiment analysis can also deliver more objective results from customer reviews, and real-time monitoring for brand reputations. These tools provide the insights needed to improve customer support, build a stronger presence, or conduct competitor research. In fact, sentiment analysis is commonly used in market research, public relations, reputation management, competitor research, stock analysis and financial trading, customer experience, product design, and many more fields.
You can deploy sentiment analysis to:
measure brand awareness and popularity
track user acceptance of new products or features
gather feedback on products and services
assess campaign effectiveness
react to complaints in real-time
research your customer service experience
For instance, sentiment analysis can inform content creation by identifying trending topics or sentiments among target audiences, guiding marketers in crafting relevant and resonant messaging. In social media management, sentiment analysis enables real-time monitoring of brand mentions and sentiment trends, empowering brands to promptly respond to customer feedback or mitigate potential reputation risks. Moreover, in customer relationship management, sentiment analysis helps identify patterns in customer sentiment, enabling personalized engagement strategies that foster stronger relationships and loyalty.
But keep in mind: just as context is key in real-world conversations, so too does context matter for sentiment analysis. These AI-driven tools may not pick up on irony or sarcasm, might not understand colloquial or idiomatic language, and may miss subtle nuances in feedback. Data analysts who design these tools must think creatively to build and update engines so they remain useful and reliable.
The Future Horizons of Sentiment Analysis
Future trends and innovations in sentiment analysis are poised to revolutionize the way businesses understand and interact with customer feedback. One significant advancement is sentiment analysis for multimedia content, which extends beyond text to analyze images, videos, and audio clips. This capability enables businesses to extract valuable insights from visual and auditory content shared on social media, online forums, and review platforms.
Additionally, sentiment analysis is expanding to encompass multiple languages, facilitating cross-cultural understanding and global market insights. As businesses increasingly operate in diverse linguistic environments, the ability to analyze sentiment in different languages becomes crucial for accurate and comprehensive feedback analysis.
Moreover, sentiment analysis is evolving to adapt to emerging digital platforms and communication channels. With the proliferation of new social media platforms, messaging apps, and online communities, sentiment analysis tools are being developed to analyze sentiment across these diverse platforms. This allows businesses to stay attuned to customer sentiments expressed in various digital contexts, enabling proactive engagement and response strategies.
And lastly, advancements in machine learning and natural language processing techniques continue to enhance the accuracy and sophistication of sentiment analysis algorithms. These innovations enable sentiment analysis tools to discern nuanced emotions, identify contextual cues, and deliver more actionable insights for businesses.
The Bottom Line: You Need Sentiment Analysis!
Sentiment analysis marketing helps businesses in gaining deeper insights into their customers, enhancing customer experiences, and bolstering their brand reputation. Deeper learnings from audience insights through sentiment analysis can lead to product and service innovations, innovations that show customers you know how to address their unmet and ever-evolving needs.
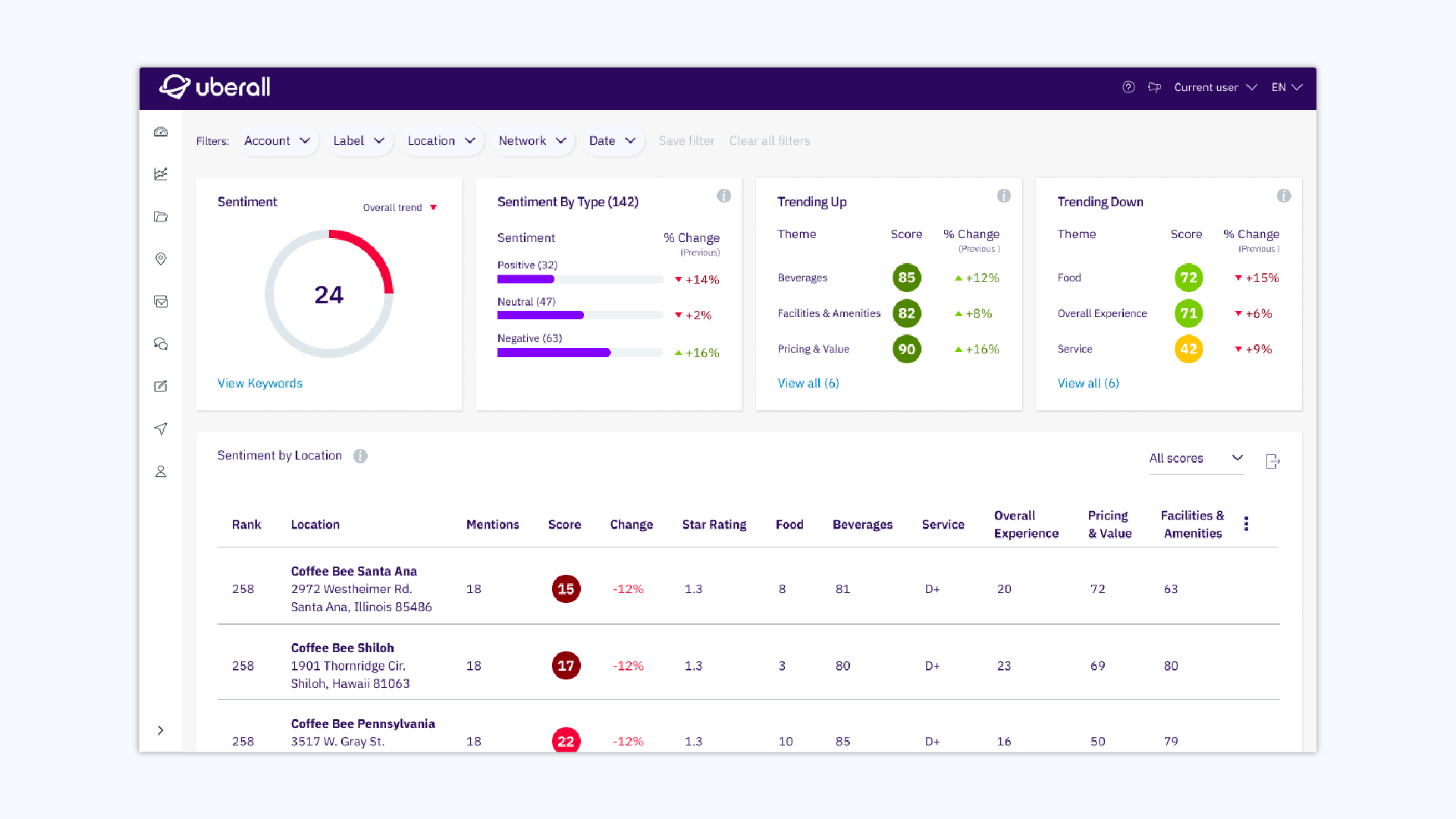
In fact, 73% of your customers expect you to understand their needs, and 63% expect that you can even anticipate them: Good business always builds on meeting customer needs with unique solutions. Showing you listen actively to customers gives marketers the opportunity to turn dissatisfied or disaffected customers into loyal supporters, while responding to the positive feedback can lead to higher customer retention.
By the way: did we mention that sentiment analysis is built into our location marketing platform? Gain full control over your reputation, and simplify reading, managing, and analyzing local reviews across the web from a single AI-powered platform.
Go Beyond Just Reviews and Ratings