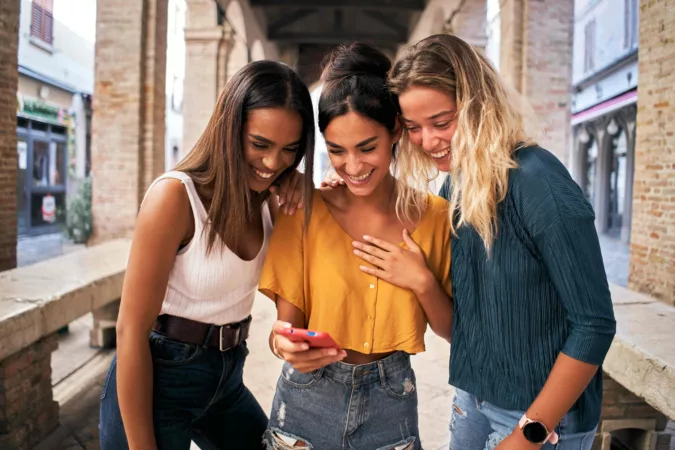
Choosing the Right Sentiment Analysis Tool for Your Marketing Needs
What would you do if you could understand exactly what your potential customers were thinking? How would you use those sorts of data insights? The most intuitive response: target your marketing messaging or digital advertising—while managing a business’ online reputation is often overlooked.
With always-on digital tools, you can respond to a potential issue emerging at one of your outlets, before it hits the media. And we all know: acting quickly to mitigate risky discussions can take less resources than responding after the fact with crisis PR to repair your brand reputation—if you catch things in time. Enter the world of sentiment analysis datasets.
In our recent introduction to sentiment analysis, we talked about how sentiment analysis software looks at what people are saying online about a topic, such as a business or a particular outlet. These tools use natural language processing (NLP) algorithms to determine the opinions, judgments, or emotions behind the “natural language” in posts.
Who Uses Sentiment Analysis Tools?
Businesses across every sector can gain data insights through opinion mining and text analysis, but service industries might benefit most immediately—locations involving constant, personal customer interactions, with operations that invite clients to review them or benefit from encouraging customers to post their experiences on social media.
Multi-location marketers seeking to make the most of customer feedback deploy sentiment analysis software to gain insights that inform local marketing movements or even company-wide business decisions—which is why sentiment analysis tools prove useful for local SEO strategies, too.
Understand how messaging lands with potential customers, enabling tweaks to the tone of voice and word choices to influence better outcomes
Spot potential issues at a specific location or outlet before they become a major problem, opening up time to tackle urgent events head-on
Use emotion detection to inform potential new programs or campaigns, and draw in more customers, or reinforce loyalty among people who already love you
Target digital advertising to encourage visits at a better pace
To sum up, sentiment analysis tools offer automated processes, saving the time and effort of analyzing and decoding every point manually.
Navigate Success Through Data-Driven Marketing
Under the Hood: How Does Sentiment Analysis Work in Practice?
Today’s sentiment analysis tools build on NLP and machine learning, riding the wave of artificial intelligence tools for more efficient enterprise operations by bringing granular data insights into business intelligence.
NLP models are trained on large volumes of text available in the public domain, like social media posts or comments and reviews, as well as company data such as chat transcripts from customer support. Then, sentiment analysis tools scan texts in question to determine attitudes based on the language (and emojis) used.
Social listening involves monitoring online conversations and discussions about a brand or topic across various platforms, aiming to understand public opinion and gather feedback. Sentiment analysis specifically focuses on analyzing the emotional tone of these conversations to determine whether they are positive, negative, or neutral, providing insights into customer perceptions and attitudes.
Analyzing at scale makes it possible to uncover customer emotions in textual data affordably. Creating your own sentiment analysis software in-house, on the other hand, would entail incredible costs. Did you know that OpenAI co-founder Sam Altman pegs the cost of training their models at more than $100 million, while keeping up continues “getting more expensive.”? Thankfully, Uberall’s platform gives you access to a quality pre-trained machine learning tool ready to perform sentiment analysis on your behalf.
Preparing the Machine Learning Model for Text Analysis
Essentially, NLP technologies train algorithms to understand text in similar ways to human brains. This starts with preprocessing, cleaning raw text data in preparation for machine learning.
In the case of NLP algorithms, the machines need some initial context for raw text data containing real human language needs. Sentiment analysis engines often begin with a process of tokenization, breaking down training texts into smaller units, then turning grouped units into representational vectors, through a process known as embedding. Machines also convert words into their root form, known as lemmatization, and filter out less-useful “stop words”.
Opinion Mining and Text Mining
Once trained, sentiment analysis software trawls the internet seeking reviews and opinions from customers across social media platforms, blogs, and other digital platforms. All of that review generation work you put in as part of your marketing strategy comes into play here, part of the software’s process of text mining; also known as opinion mining.
The sentiment analysis software finds reviews and gathers them together for deeper digging. It can then parse that large volume of text, and so move onto the next phase of the process: analysis and scoring.
Text Analysis, Emotion Detection, and Sentiment Scoring
Once the software converts raw text into an analyzable format, it moves onto seeking out keywords for analysis. Extracted keywords receive a sentiment score based on a measured scale that indicates emotional elements built into the trained model.
Many NLP algorithms will perform text analysis to assign sentiment scoring. These look for terms like “brilliant”, “great”, “ok”, “hate”, “disgusting”, and so on, to label content as “positive”, “neutral”, or “negative”.
Sentiment can involve analysis through a number of methods:
Graded sentiment analysis offers polarity precision, such as interpreting starred reviews on a scale of good to bad.
Emotion detection allows you to go beyond detecting emotions, depending on the lexicon or complex machine learning NLP algorithm the software trained on.
Aspect-based sentiment analysis (ABSA) can zoom in on particular features of your products or services, such as customer service or cleanliness.
Intent analysis checks to see whether users appear interested in purchasing, or simply browsing.
The emotions assigned through sentiment scores depend on rules-based systems selected for training each model. Because initial selections for scoring and lexicon build out the sentiment classification assigned in training, those choices influence results significantly.
Effective sentiment analysis software aggregates processed data for high-level views while enabling deep dives into their text analysis, to highlight individual issues or emotions of potential interest. Because they continue to trawl, users can interact with findings in real-time, a feature especially useful for navigating PR crises. Automated machine learning sentiment analysis can also provide options for instantly alerting the right teams for resolving an issue.
"I highly recommend tracking and reporting on all aspects associated with reviews, including NPS and sentiment analysis trends over time to understand how real-world customers are experiencing your business, whether customer experience is yielding referrals, and where the business can improve to retain existing customers and earn new ones."
The Features to Keep in Mind for Sentiment Analysis Software
Ready to look beyond a simple count of mentions or comments? You need access to consistent and accurate data, a key factor to consider when choosing local SEO services—because not all sentiment analysis tools are created equal. Consider whether you need certain sentiment analysis features to:
overall sentiment across a specific time period or search term
accumulated sentiment over time, highlighting spikes and troughs
brand sentiment, or sentiment by topic
granular details, such as who’s posting mostly positive or negative
trending conversations and trends in conversational activity
keyword clouds for the most frequently used words in relevant reviews
You might also want to consider how you want to receive data insights: what kinds of visualization would serve your needs best? How customizable can you make your analysis? Does this particular tool allow you to interact and operate in real-time? How does that tool integrate the data for analysis—what platforms can it monitor, and how does it connect to them? And how user-friendly is the interface? Is color-coding available? After all, you need to work with the results!
Best Practices with Sentiment Analysis Software
When incorporating sentiment analysis into your local marketing strategy, beware the pitfalls: NLP algorithms do not always detect sarcasm or deal with ambiguity, though results continue to improve. Even so, from a birds-eye view, these useful tools monitor overall brand health, spot reputation crises early, track campaign performance, perform competitor analysis, and improve customer care.
Sentiment analysis software should not act alone: combine automation with proactive reviews and listings management for effective synergies, as KFC found. While focusing on increasing their visibility and appeal to local customers, KFC listens to customer preferences more and more, serving them targeted advertising at the right time and place to make the most of their munchies.
Or look to the success of sporting goods store Decathlon, who worked with Uberall to optimize multiple processes, improving their positioning and generating increased digital traffic. The AI-powered platform enabled Decathlon's marketing and customer service teams to extract important data insights more efficiently, giving them live views into customer preferences and behaviors.
AI-Powered Sentiment Analysis for Multi-Location Marketers
Marketing technology has finally caught up with the needs of multi-location marketers, harnessing machine learning algorithms and artificial intelligence to deliver game-changing tools that transform customer feedback and emotions into data insights that can drive timely business intelligence.
Sentiment analysis software can provide the key to increasing engagement and visibility online by getting to know your customers and prospects all the better. By understanding their motivations, their emotions, and their behaviors, multi-location marketers can generate content and campaigns that find customers exactly where they are, and where they need to be.
Uberall’s multi-location marketing platform offers plenty of functions to help your brand show up and stand out when nearby customers search. Sentiment analysis and data insights sit within the review management module, which includes an AI-powered review assistant for auto-responding to reviews in any language, and a response library to hold templates for your teams, so they can reply quickly while staying on-brand. These insights take you to the “why” behind ratings and continue improving them.
Learn How Uberall Can Boost Your Multi-Location Business